About 2 months ago, I had arrived at the office at about 8:45am, sat at my desk and was about to start my day. The only problem was that I, for the life of me, could not remember my password. You see, this is a bit laughable because for the past year or so, I had typed in that exact password, sometimes from muscle memory. I had done it at the beginning of that week, all the days before, and even late the previous night.
But in that moment I couldn't even remember the first letter. After about an hour, taking a break, missing a morning meeting, getting locked out (a Mac will do that after several failed attempts), I did get back in.
This wasn't just about forgetting a password. It was a wake-up call – a small but telling sign of cognitive overload. As I sat there that morning, staring at my login screen, I realized this memory lapse was symptomatic of something larger: the mental toll of trying to keep pace with the relentless advancement of AI. You see, that period, like most of the year, had been marked by lots of effort to stay current – tracking breakthrough papers, implementing new models, adapting to paradigm shifts, all while maintaining regular work responsibilities. And I wasn't alone. In conversations with colleagues across the AI research and engineering space, a pattern emerged. While we all shared genuine excitement about being in the field during this revolutionary time, there was also an undercurrent of exhaustion – a particular kind of fatigue that comes from trying to match the unprecedented pace of AI advancement.
This is what I've come to think of as "AI Fatigue."
Note: This post is more of a personal reflection, than other more focused AI/Agents posts. It’s inspired by some recent events. Bear with me.
Podcast Audio is generated using NotebookLM1
AI Fatigue - What is it?
AI fatigue is the collective exhaustion experienced by individuals and organizations in response to the unrelenting pace of AI advancement. It reflects the mental, emotional, and operational toll of trying to adapt to an unprecedented rate of change that has sustained for a relatively long period (24+ months) with little/no signs of slowing down.
In some way, it's an acknowledgement that the pace of AI is gargantuan2 and adapting to it has costs that we must all be clearly aware of. The duration of this rapid pace has also been gargantuan - the release of ChatGPT (~ 24 months ago, Nov 2022) marked the beginning of this period .. that sometimes feels like many, many years.
And it is new territory for the humans of AI, and organizations too.
The Three Firehoses of AI Progress
When I say the pace is gargantuan, what do I mean? Here are some examples:
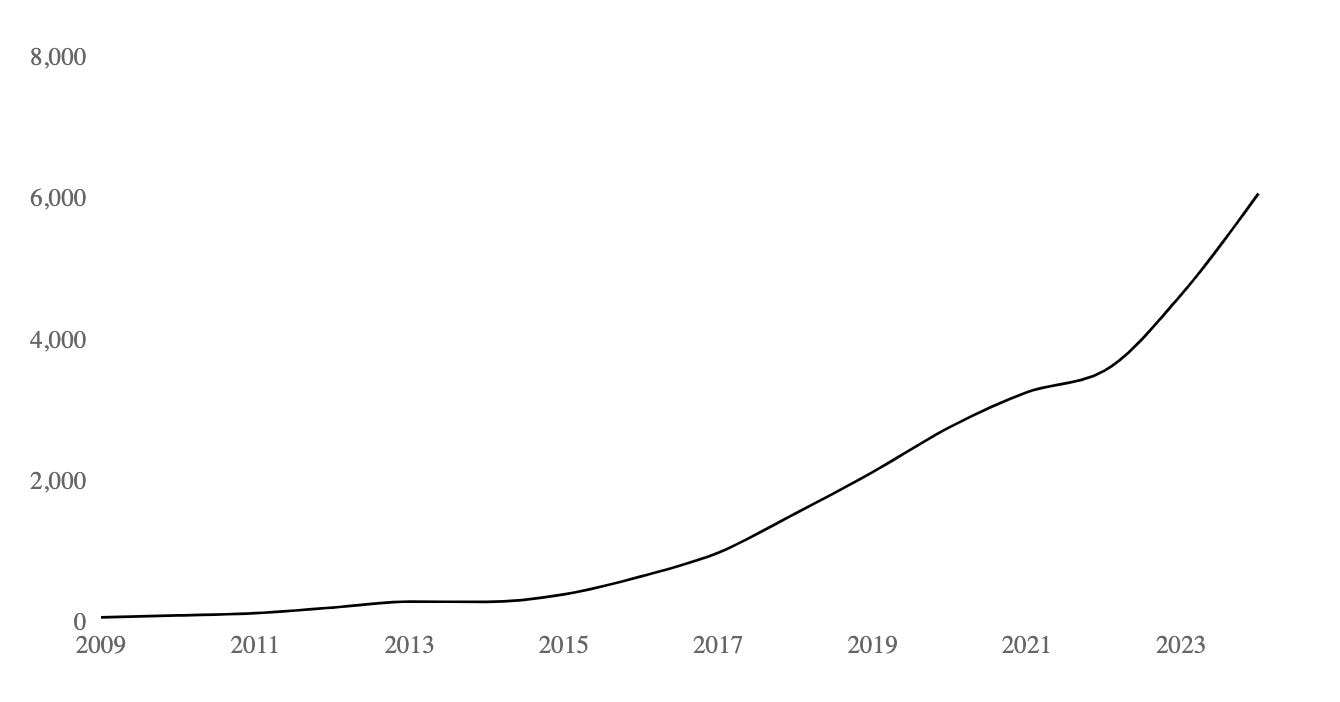
The Research Firehose.
There were 21206 submissions on arXiv in just December 2024 (the data is all here). If we focus on AI related sub categories (Computer Vision, Machine Learning, NLP, Robotics, AI, and Information Retrieval), there were:~6,052 papers/month.
~1,513 papers per week
About 288 papers per day on average
If you skimmed the papers (~10 mins per paper) and you had about half a day (4 hours) each day, you’d only cover 8% of all papers. Assuming you follow some website or twitter account to help you find that 8%.
The Model Firehose
New models seem to be released every other week. Several big ones every other month (OpenAI, Claude, Gemini updates, see just how much was announced in the last two months alone from Google / OpenAI / Anthropic). Plus the 1.2 million and growing smaller models on HuggingFace.The Announcement Firehose
Models advances seed a series of a broader ecosystem of startups, tools, etc leading to further announcements and updates. This also includes all the AI hardware stuff that I have not really kept up with (Semianalysis does a good job of this).
Hidden Costs of Adaptation Drive Fatigue
Actors in any AI-adjacent field (researchers, engineers, teams) must constantly adapt to the field's rapid pace. In many cases, adaptations aren't optional and demand significant investment of time and resources. This constant need to respond is a key driver of AI fatigue.
Some examples of how adaptation costs play out:
Disrupted Research Cycle
Researcher have to constantly update their work to cite/integrate new papers. In some cases, it means abandoning or significantly updating projects - as they become no longer relevant or completely scooped by new work.
Accelerated Engineering/Product Cycles
Engineering teams having to constantly update entire systems, facing endless sprints to update existing projects each time new models are released or fundamental changes occur
Disruption in Other Fields
Many fields have been or will be disrupted in some way as ChatGPT and its siblings have made their way into the world. An example is that educators must now update their approach to teaching and evaluation in a post-GenAI world.
Moral and Societal Responsibility
Just a few years ago, AGI seemed like distant science fiction – the sort of hubris that earned eye rolls from serious researchers. Today, it looms as a tangible possibility, demanding deep reflection from everyone in the field. This shift brings with it an enormous responsibility. There is a chance that we are potentially shepherding a transformation that could fundamentally reshape human society (big claims, I know!).Engineers and researchers who once focused purely on technical challenges now find themselves grappling with philosophical questions and wrestling with societal implications. Questions that were once abstract thought experiments have become pressing practical concerns: How will this technology impact human labor? What safeguards need to be in place? How do we ensure the benefits of AI are distributed equitably?
Overall, the costs increases with proximity to the field.
AI researchers and engineers > AI adjacent industries > AI disrupted industries > others
As we enter into an age of AI agents, this might generate even more wider reaching costs (AI slop, Agentic Noise). Perhaps a post for a different day.
Depleted Surge Capacity
So, what is the impact of all of this? How is this "tiredness" felt?
During the pandemic, I read an insightful article by Tara Haelle titled "Your 'Surge Capacity' Is Depleted — It's Why You Feel Awful." The article introduced me to the concept of surge capacity, which is a collection of adaptive mental and physical systems that humans draw on for short-term survival in acutely stressful situations.
In my opinion, this concept resonates strongly with what we're experiencing in the AI field today.
Surge capacity3 is a collection of adaptive systems — mental and physical — that humans draw on for short-term survival in acutely stressful situations. But natural disasters occur over a short period, even if recovery is long. AI advancement is different — the surge demands seem to stretch out quite a bit into the near future.
Surge capacity is designed for short-term emergencies - natural disasters, acute crises - where there's typically a clear end in sight. But just as the pandemic stretched on indefinitely, the current pace of AI advancement shows no signs of slowing down. As humans, it is challenging to maintain 150% effort for 18 months straight when our surge capacity systems are designed for sprint scenarios, not marathons.
The Cost / Impact of AI Fatigue ?
The impacts could manifest in several key ways:
Individual Impact
Cognitive Load and Context Switching:
The constant need to stay updated while maintaining regular work creates significant mental strain. Engineers and researchers must frequently context switch (I personally find this to be brutal, and counter productive) between keeping up with new developments and focusing on their core work.Psychological Impact:
There's a persistent feeling of being "behind" despite working at maximum capacity, leading to anxiety and imposter syndrome. This is especially challenging for those used to being at the cutting edge of their field.Disrupted Create/Consume Balance:
As an engineer, I feel fulfilled when I create at least just as much as I consume. In this fast paced world it is easy to spend so much time adapting that little time is available to create.
Organizational Impact
Sprint Fatigue
Teams are caught in endless cycles of updates and adaptations to keep pace with new models and capabilities. While I have seen many managers navigate this gracefully, it could lead also to burnout if not carefully managed.
Hype-Driven Development
Organizations face the dual challenge of maintaining existing systems while rapidly innovating to stay competitive. In extreme cases, I have seen startups, groups etc., race to adopt AI models without ascertaining first that it is the best tool to address their business problem.
Who Else Is Talking about This?
I certainly am not the first to explore this. In various ways, a few people have engaged with the subject at both the individual and organizational level.
AI Fatigue: When Innovation Feels Like Overload.
Neil Sahota (speaker and former consultant at IBM) provides an organizational perspective, defining AI fatigue as “the mental and emotional exhaustion individuals and organizations experience due to the constant barrage of information, sales pitches, and lofty AI technology promises.”.
In my opinion, his article touches on how misconceptions about AI capabilities can drive a problematic FOMO cycle - pushing organizations into adopting technology for technology's sake rather than focusing on genuine problem-solving.I sensed anxiety and frustration at NeurIPS’24
In this article, Kyunghyung Cho, a Professor at NYU reflects on how the AI/ML field is changing and how all of this creates anxiety for students about to graduate. The main thesis is that the field has transformed from one that valued open-ended research and PhD-level innovation to one that increasingly prioritizes practical implementation and product development, leaving many current PhD students caught in this transition with expectations set by the previous era.Chris Olah (Researcher at Anthropic) reflects on how AI research has evolved from a “grassroots / individual” model to a “focused bet / team science” model. He points out how the type of advances needed in AI today is better accomplished by the “focused bet / team science” model, how industry labs have successfully transitioned to this winning model and how academia has not.
- writes about changes in the field post-ChatGPT on the interconnects newsletter (Behind the curtain: what it feels like to work in AI right now (April 2023)).
“The ChatGPT spark has caused career changes, projects to be abandoned, and tons of people to try and start new companies in the area. The entire industry has been collectively shaken up — it added a ton of energy into the system” Felix Hill (RIP) has written a post on mental health and life as well as the stress of working in modern AI. He reflects on how “Doing AI research at the moment can feel like participating in a war” - a nod to how research outcomes are now closely tied to products, bottom lines and company stock price and researchers are thrust in the forefront of enterprise competition.
His posts also inspired me to publish this post after it being in draft form over the last 12 months.Several industry leaders have weighed in on the moral/societal impact of the rapid advances in AI. Some are listed below:
Richard Socher (CEO, You.com) likens the current AI wave to the Industrial Revolution, the Enlightenment, and the Renaissance combined. In his words, “It creates even more pressure on our moral frameworks and how we define our in-group vs out-group, how we merge empathy with utilitarian effective altruism.”
Sam Altman from OpenAI (and others) have discussed the concept of basic income as one way to manage potential job loss impact of AI/AGI.
“For years, Altman has been publicly worrying that basic income will become necessary as AI eliminates traditional jobs while creating huge stockpiles of wealth held by a few.”. In this 2021 article Altman writes “If public policy doesn’t adapt accordingly, most people will end up worse off than they are today.”“We believe that, in 2025, we may see the first AI agents “join the workforce” and materially change the output of companies.” Altman in his Jan 2025 reflection. He also alludes to the stress and rapid pace.
Dario Amodei (CEO, Anthropic) provides valuable context in his October 2024 essay (Machines of Loving Grace) about AI's transformative potential. He acknowledges the intense pace of change, noting that most people are "underestimating just how radical" AI's impact could be. While optimistic about AI's potential, he emphasizes that achieving positive outcomes "will require great sacrifice and commitment on all of our parts."
Conclusion
While this note/reflection has focused on examining AI fatigue, the question of solutions remains complex (and unaddressed).
I do think that traditional approaches to managing professional stress and burnout – setting boundaries, maintaining work-life balance, prioritizing mental health – apply here.
The excitement and potential of AI development remain as compelling as ever. My reflection on AI fatigue isn't a critique of this progress or the dedicated engineers behind it - rather, it's an acknowledgment of the very human experience of trying to keep pace with such rapid advancement. I'm in awe of all the incredible engineers and teams working in this space – from colleagues at Microsoft to teams at OpenAI, Anthropic, Google, and beyond.
For anyone feeling overwhelmed by this pace – you're not alone.
I find myself relating to Kyunghyun Cho's reflection..
"unfortunately i could only work my way through (partly) understanding the source of anxiety and frustration i could sense from these immensely brilliant students but cannot think of a way to help alleviate such frustration. after all, it looks like i may have greatly but unintentionally contributed to the situation that makes them frustrated and anxious about their careers and future. sorry!"
Perhaps the first step toward managing AI fatigue is simply acknowledging its reality – and giving ourselves permission to discuss it openly, seek support, and work toward more sustainable practices in this rapidly evolving field.
Thanks for reading. And yes, I see the irony talking about fatigue and then writing about it.
Needless to say here, views here are my own.
NotebookLM - https://notebooklm.google.com/
Enormous. Did I have to use an unusual word here? Maybe?
Speaking of Psychology: The role of resilience in the face of COVID-19, with Ann Masten, PhD
Share this post