How will AI Impact Academic Research (and Publishing) by 2027?
#39 | A thought experiment on how advances in strong AI could completely drive the scientific research process in the near future.
Here’s a thought experiment:
It's 2027 - and the academic research landscape has completely devolved into a battle of AIs - only those with the strongest AIs win.
Academic papers are written with or by AI. Reviews are conducted by AIs. Only teams with AIs superior to the reviewer AIs pass the review process and get published.
Research departments are forced to invest increasing slices of their resources in obtaining superior AI because nothing else really matters. The innate joy of discovery, the toil of a PhD, the glory of paper acceptances, the ups and downs - all gone or changed. Across the world, many groups simply do not have the financial resources to obtain these advanced AIs and are immediately uncompetitive. Even more do not realize this is now how the system works (all they see is their dwindling publication hit rate).
The previous prestige or meaning of being published in a top conference is destroyed. Many academic institutions stubbornly refuse to acknowledge this (it is extremely problematic for them, as academic reputation has been built on some version of papers per faculty; they have not much choice), but the rest of the world can see it clearly - an emperor's new clothes situation.
Research and publications still exists, but it has fundamentally and irreversibly changed.
How We Get There
We do not arrive at this overnight. It's been a slow arc across several stages:
Pre-2022 | The Elite University Advantage
Top research venues are dominated by elite university research groups and their students or extensions of similar groups working at industry research labs. They have the best scientists, strong English writing skills needed for publications, extensive experience with the review process (there are rampant tales of how certain "Stratford professors" can edit a shitty paper and turn it into gold... they have the magic touch), and the resources to run the best experiments - user studies, GPUs for computation etc. The primary research publication edge comes from adjacency to these groups.
2022-2025 | GPTs Level the Playing Field (Somewhat)
ChatGPT begins to level the playing field in academic publishing. There are early reports of sparks of artificial general intelligence (AGI) observed within AI models. Non-native English speakers can now produce adequate prose, though not yet matching the magic of "Stanford professor" level of polish. Researchers who use these tools judiciously improve their publication chances, while discussions emerge about the concerning trend of papers containing entire sections of mindlessly (AI) generated content. The volume of submissions skyrockets, leaving conferences and journals struggling to manage the influx.
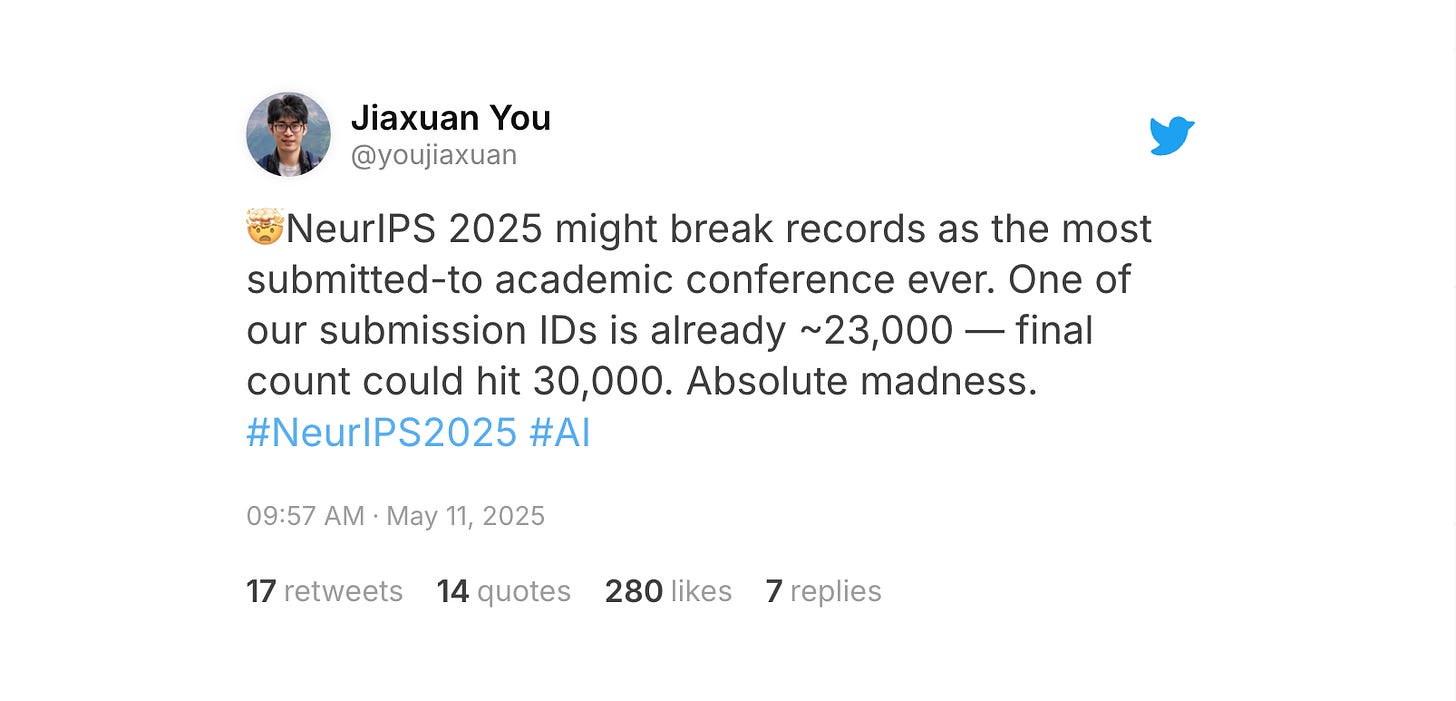
In the latter part of this phase, AI-enabled research experiences a dramatic step-function increase.
Models can now generate “Stratford professor” level prose.
Simultaneously, agentic AI systems (such as Sakana AI's "AI Scientist") demonstrate early capabilities for autonomous experimentation—a critical component of research. The academic community faces a growing challenge: distinguishing genuine, important research contributions from AI-generated material created solely to secure publications.
March 12, 2025 : A paper produced by The AI Scientist-v2 passed the peer-review process at a workshop in a top international AI conference. Source: SakanaAI
Conferences begin to buckle under the sheer load of submitted papers, many of which amount to merely incremental ideas or outright low-quality content. To cope with this deluge, organizers introduce new or expand current measures—requiring authors to declare the percentage of AI-generated content, explicitly articulate their novel contribution, and commit to reviewing other submissions. Some venues implement rigid formatting requirements, mandatory reproducibility checklists, and expanded conflict-of-interest disclosures (). Despite these well-intentioned gatekeeping efforts, the fundamental problem persists: the volume and variable quality of submissions continue to overwhelm the traditional peer review system.
2026 | The Quality Crisis and Heimdall - The AI Reviewer
Some clever YC startup - HeimdallAI - emerges from a Stratford lab. Their bright idea is to stem the flood with an automated AI review tool. Their slogan - “the guardian at the gate to deny low-quality slop”. A few million dollars later of VC funds, the first version of Heimdall is integrated into a couple of academic publishing systems. They become the first pass arbiters of papers of all papers.
At this stage, Heimdall is purely advisory. If your paper fails Heimdall, a human review only takes output into advisement and you the author get a chance at a rebuttal.
2027+ | The Research Paradigm Shift
At this point another curious thing starts to occur - AI for science is on fire!
Researchers find out that the AI of the day is so remarkable that it honestly (a very bitter pill to swallow) does research better than they can. It comes up with better ideas, has learned to leverage its broad knowledge alpha to synthesize new knowledge by connecting widely disparate fields (a known driver of discoveries/innovation).
The new AI capabilities here are possibly enabled via advances in delibrate Reinforcement Learning that instructs the AI to explore radical divergent thinking and experimentation across disparate disjoint domains towards genuine scientific discoverry
It can design and independently conduct experiments via agents especially for digital research (building software, models, iterating), simulate human studies (social science, psych, HCI). Advances in automated robots are still behind, available robots are still prohibitively expensive, so physical experiments can not be explored similar to digital experiments ... so there is somewhat limited fallout on the physical sciences.
Legitimate breakthroughs now result from a situation where the researcher sits and watches the AI work with some feedback and requests for explanations here and there. The same happens on the AI reviewer side.
An important note here is that the delegation of research production and review to AI will likely not be voluntary. It will likely be due to a humbling realization that the AI is better, faster and cheaper and the researcher’s time is better spent “supervising” the work rather than trying to do the work.
HeimdallAI creators have also been extremely hard at work. They announce that Heimdall is bigger, stronger, better, faster. It is excellent at adjudicating what is low quality, with evidence, what is slop, what is genuinely new, what is incremental. It can scan every knowledge base, utilize its training data (which is all available human data at this point), verify its work. Extensive experiments are conducted and 95 out of 100 times, human reviewers agree with it and in the 5% where humans don't agree, it is subjective enough that it is hard to say it's wrong.
Across these eras, three key metrics evolve dramatically (see diagram above):
Publication volume rises exponentially as AI lowers creation barriers. Starting at medium levels pre-2022, it reaches extremely high volumes by 2027+ when AI conducts research autonomously, saturating the academic ecosystem.
Research costs increase steadily as the AI arms race intensifies. Despite initial democratization of capabilities, by 2027+ only well-funded institutions can afford cutting-edge AI systems necessary for competitive research, creating new inequities.
Acceptance rates (for the average researcher) follow an inverted U-curve - rising briefly during AI-assisted writing (2022-2025) before plummeting as AI reviewers become gatekeepers. Quality standards become determined by AI-to-AI competition rather than human expert judgment.
These intersecting trends reshape academia: more submissions, higher entry costs, and lower acceptance rates transform research from a human-centered creative process into a resource-driven AI capability arms race.
The Fallout
AI Arms Race in Academia
AI research effectively is reduced to "my AI is better than yours". Only groups with the best AI pass Heimdall. Beyond the elite labs, research groups across across North America without the millions needed don't publish at the best venues. Teams in Africa, South East Asia and developing countries in general - same story but larger impact.
Decline of Research Motivation (and Expertise)
Few researchers are motivated to build up skill equivalent to the AI. Extremely difficult. And for what? Only intrinsically motivated researchers keep the torch of deep mastery alive.
Crisis of Academic Credibility
The publication game is no longer tenable. Publications used to be a measure of accomplishment or renown. But now that AI does the work, what does this even mean? Academia struggles to keep the ruse going but the rest of the world can see. New metrics for reputation emerge - from citation count to software download counts.
The Resistance Movement
The resistance is forming in unexpected places. Some universities create "AI-free" tracks and journals that require rigorous verification of human authorship. Underground conferences emerge where researcher reputation comes from proving they can reason through problems the AI cannot or build usable prototypes that solve problems as evidenced by verifiable usage metrics - downloads, human reviews, MAU? etc.
The Meta-Research Paradox
A black market for "Heimdall exploits" develops - methods to trick the AI reviewer into accepting work that shouldn't pass. Passing Heimdall becomes a target that must be ruthlessly optimized (Goodhart’s law). Ironically, these exploits themselves become the subject of papers, creating a bizarre meta-research ecosystem.
Alternative Communication Platforms
With traditional publishing losing meaning in an AI-dominated landscape, researchers may naturally shift toward alternative communication channels. Platforms like Substack blogs and open-source repositories (GitHub, GitLab) offer direct paths to share findings without the AI review gatekeeping. While AIs excel at generating papers, they haven't reduced the effort required to create genuinely useful artifacts and working systems. This reality suggests a potential shift where demonstrable, functional contributions gain prominence over paper credentials.
Wait - What? What are the Consequences?
The grim scenario I've painted is a thought experiment and written in a manner that reads like sci-fi - I know. But it also a genuine call for reflection and discussion.
Academia today is already somewhat broken - "publish or perish" culture has created a flood of incremental papers, many of which are optimized to navigate reviewer preferences (or other incentives like graduation, tenure, academic jobs etc) rather than solve meaningful problems. Academic incentives reward citation counts and publication numbers, as opposed to actual real-world impact.
What AI brings is not a new problem but an extension of the existing one. The publication game shifts from "how many papers can you produce" to "how powerful is your AI?" As models continue to advance, we face profound questions about the future of academic research:
What happens when publication success becomes an AI arms race? AI-enabled research will likely produce genuinely great research, but access to these tools becomes the primary determinant of success, creating a system that favors the well-funded and technically equipped.
What remains of the researcher's craft? The joy of discovery, the satisfaction of solving problems, the intellectual growth of a PhD - all potentially hollowed out when AI can generate better (yet to be seen) ideas and execute experiments more effectively than humans.
How do we address the inequalities? The gap between resource-rich and resource-poor institutions widens dramatically when cutting-edge AI becomes necessary for publication success.
What will a publication even mean? When AI generates the ideas, designs the experiments, and writes the paper, the credential itself loses meaning - though academia may be slow to admit this.
These aren't distant concerns - they're already emerging. The fundamental question is whether we proactively reshape academic practices to preserve what's valuable about human research in an AI-augmented world, or whether we drift into the dystopia I've described.
What aspects of research are uniquely human and worth preserving? That's the conversation we need to have - before it's too late.
This writeup in part is inspired by
’s piece on the need for discussions around the economic impact of AI, and work by Kokotajlo et al (AI 2027) on gaming out negative outcomes (end of humanity) of superhuman AI.
Limitations and Caveats:
There are a few limitations to this article that I am acutely aware of. First, I assume that AI will indeed get better at research than human researchers without presenting (there are arguments for this being unlikely).
In my opinion, some arguments in favor are:
The scientific method (and individual research processes) is well-structured and, contrary to popular belief, follows patterns (hypothesis generation, experimentation, analysis, reporting/writing).
My simple argument is that AI training mechanisms are well-suited to domains that have discernible patterns and for which digital examples and feedback loops (for reinforcement learning) exist.Advances in reinforcement learning will get us closer to solving the “AI cannot discover new things”. Today autoregressive model training objectives “limit” the ability models to explore cognitive leaps - think outside the box in solving problems by connecting patterns across disparate domains. If we can represent this type of behavior as RL training data - we can solve this problem.
Second, I haven't sufficiently emphasized or championed the creative spirit of the research community, nor have I fully explored the innovative ways researchers will likely respond to these challenges. I am hoping discussions (like this article) will set us all up for success. After all, I am a scientist myself—and will likely be on both sides of this equation, using AI for research while also helping build systems like the hypothetical AI Reviewer - "Heimdall".
I welcome further discussion on both of these points, as they represent important dimensions of this complex issue.
Hmm… I see a role for humans producing meta research. Or simply research on a higher level of abstraction, interpreting what the AI generated research really tells us. I doubt we will move into a world where all of us just blindly accept AI outputs.